Data Mining and Functionalities of Data Mining
- techskill065
- Dec 23, 2021
- 2 min read
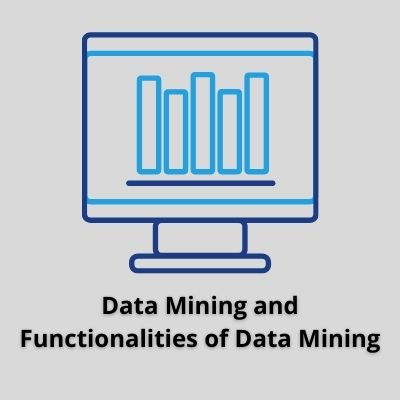
Data mining is the process of sorting through large data sets to identify patterns and connections that can help break business problems through data analysis. Data booby-trapping ways and tools enable enterprises to prognosticate unborn trends and make further- informed business opinions.
Data mining is a crucial part of data analytics overall and one of the core disciplines in data wisdom, which uses advanced analytics ways to find useful information in data sets. At a more grainy position, data mining is a step in the knowledge discovery in databases (KDD) process, a data wisdom methodology for gathering, processing and assaying data. Data booby-trapping and KDD are occasionally appertained to interchangeably, but they are more generally seen as distinct effects.
Functionalities of Data Mining
Following are the functionalities of data mining -
· Classification
· Association Analysis
· Cluster Analysis
· Class/ Concept Description Characterization and Discrimination
· Prediction
· Outlier Analysis
· Evolution & Deviation Analysis.
· Classification
It uses data models to predict the trends in data.
This path assigns the alphabet in data sets to different orders defined as piece of the data mining process. Decision trees, Naive Bayes classifiers, k-nearest neighbor and logistic regression are some exemplifications of classification approaches.
· Association Analysis
In data mining, association rules are if- also statements that distinguish connections between data basics. Support and confidence standards are used to exact the connections-- support means how constantly the affiliated basics appear in a data set, while assurance reflects the number of times an if- also statement is correct.
· Cluster Analysis
Unsupervised category is called cluster analysis. It's correspondent to the category where the data are grouped. Unlike category, in cluster analysis, the class label is unknown. Data are grouped grounded on clustering algorithms.
· Class/ Concept Description Characterization and Discrimination
When you sum up the general features of the data, it's called data characterization.
It compares the data between the two classes. Generally, it maps the target class with a predefined group or class. It compares and contrasts the characteristics of the class with the predefined class using a set of rules called discriminant rules.
· Prediction
Prediction finds the missing numeric values in the data. It uses retrogression analysis to find the unavailable data. However, also the prediction is done using classification, If the class tag is missing.
· Outlier Analysis
Outliers are data basics that can not be grouped in a given class or cluster. Also known as challenges or surprises, they're frequently genuinely important to identify.
· Elaboration and divagation analysis
Elaboration and divagation analysis belong to the study of time related data that changes in time. Elaboration analysis models evolutionary trends in data, which assent to characterizing, comparing, classifying or clustering of time related data. Divagation analysis, on the other hand, considers disputes between measured values and anticipated values, and attempts to find the reason of the diversions from the anticipated values.
I hope like our blog on data mining and functionalities of data mining.
Comments